Towards virtual screening of chemical products for sustainable formulation development
Foods, consumer products, coatings, and inks are formulated to exhibit desired properties – stability, shelf life, texture – by controlling their chemical composition and microstructure. Behind their apparent simplicity, formulated products are complex, multicomponent, multiphase materials, comprising a multitude of ingredients. Because of this complexity, the effect of each ingredient on stability, processability, performance, and user perception is difficult to predict a priori. The formulation industry is facing this challenge while striving to accelerate the screening of new formulations. The development of predictive models, including machine learning methods, and rapid screening methods will facilitate the replacement of ingredients of petrochemical origin and the introduction of biobased ingredients, thereby supporting the transition to a circular economy.
Formulating for sustainability
Formulated products – which include house and personal care products, pharmaceuticals, foods and drinks, adhesives, paints and inks – are complex mixtures of several ingredients, carefully developed to achieve desired texture, stability, and performance in applications. Ingredients include polymers, nanoparticles, surfactants, solvents, and active ingredients (Figure 1). The delicate balance of their intermolecular interactions determines the microstructure and performance of the product, and changing a single ingredient is often detrimental.

The design and development of new products – in response to fast-changing customer demands and increasingly tighter regulatory frameworks – can require several years, with significant R&D costs and frequent failures. The scale of the problem is significant: beauty and personal care products alone correspond to a market valued at $435 billion in 2020 (CAGR 4.35% between 2021 and 2026).
The formulation industry is currently faced with the challenge of making consumer products safer and healthier, while maintaining their performance, in response to the 2020 ‘Chemicals Strategy for Sustainability Towards a Toxic-Free Environment’ of the EU, as well as to increased consumer awareness. The formulation of new products, or re-formulation of existing ones, also underpins the energy transition and the protein transition.
For example, the replacement of ingredients (typically surfactants and polymers) with bio-based alternatives is a growing challenge as we transition away from ingredients of petrochemical origin. Similarly, because food products made from plant-based proteins are currently designed to exhibit comparable properties to animal foods in order to promote consumer acceptance, extensive optimization of new food formulations is required. Bans on ingredients that pose environmental or human health hazards also prompt their replacement and the redesign of formulations. For example, in 2017 the EC proposed the ban of two siloxanes (D4 and D6), which are now in the ECHA Candidate List.
The chemical industry is rapidly adopting new approaches to accelerate the formulation and the re-formulation of products
This regulatory change can have a significant impact, considering that these silicones are used in hundreds of products, all of which would have to be reformulated with safer materials such as biopolymers. Similar situations arise every year when new ingredients are added to the ECHA Candidate List and ECHA Authorization List.
Given the costs and effort required, the chemical industry is rapidly adopting new approaches to accelerate the formulation and the re-formulation of products. To this end, new analytical methods for high-throughput or rapid screening of formulations, and predictive models for virtual screening and in-silico product development, hold significant promise.
Modelling approaches for virtual screening of formulations
Several modelling approaches are available to predict product properties from chemical compositions and simulate manufacturing processes. Different modelling approaches are appropriate for different length scales relevant to product development (Figure 2) and depending on the quality of available data.
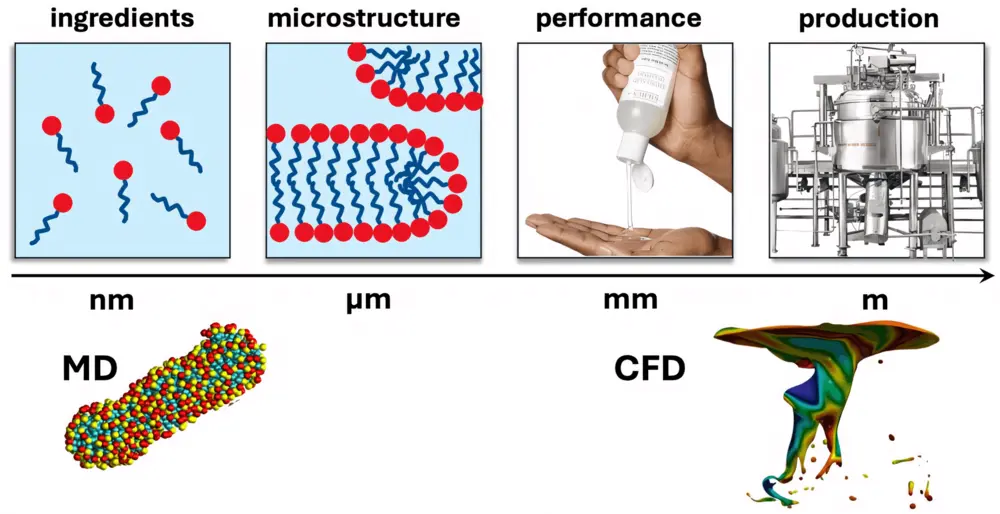
MD of surfactant micelle reproduced from J. Chem. Phys 143, 024905 (2015). CFD of stirred vessel reproduced from Chem. Eng. J. 472, 144807 (2023)
Design of experiment (DOE)
Empirical models that correlate formulation compositions with desired properties are often used in combination with design of experiment (DOE) approaches to systematically explore the effects of different components on product performance. This approach is advantageous for its relative simplicity, and can be valuable for initial formulation screening, but doesn’t provide new mechanistic insights. As such, it does not guide the development of new design principles that can be later adopted in other formulation or re-formulation projects.
Multiscale chemistry simulations can provide very detailed insights into molecular interactions and self-assembly processes
Density functional theory (DFT) and molecular dynamics (MD)
Mechanistic modelling approaches can accurately capture the underlying physical, chemical, and thermodynamic mechanisms governing formulation behavior, but also come with a set of challenges depending on the length scale. At one end of the range of length scales, multiscale chemistry simulations, including density functional theory (DFT) and molecular dynamics (MD) simulations, can provide very detailed insights into molecular interactions and self-assembly processes. These predictions can support virtual screening of new formulations in an early stage of development. However, the prediction of macroscopic properties, for instance viscosity, remains challenging due to the mismatch in length and time scales between the simulation results and the available experimental data.
Computational fluid dynamics (CFD)
At the other end of the range of length scales, computational fluid dynamics (CFD) simulations can be used to study flow and mixing processes in formulations. The prediction of CFD models applied to product performance or process design requires accurate estimation of model parameters. Given the complexity of formulations, their properties are very sensitive to flow conditions, and need to be characterized in process-relevant conditions, which is not always possible because process flow conditions can be outside the range accessible to analytical equipment.
Therefore, at both ends of the range of length scales, the bottleneck lies in the availability of high-quality data either for estimating model parameters, or for the validation of the model predictions. This bottleneck will not be overcome by only improving computational power or accuracy of the models, rather it requires the simultaneous development of analytical methods that can help bridge the current gap.
Artificial intelligence (AI) and machine learning (ML)
Finally, artificial intelligence (AI) and machine learning (ML) are emerging as powerful techniques for modelling and optimizing chemical product formulations. These approaches require large, high-quality datasets to identify patterns, correlations, and optimal formulations. Data-driven optimization algorithms can enable efficient exploration of formulation design space, but their success depends on the quality of the training data, and on robust validation procedures. Also here, the development of analytical methods that can provide sufficiently large and high-quality training datasets is key to unlocking multi-fidelity, predictive tools that combine mechanistic models with data-centric approaches and are capable of reliable uncertainty quantification.
New experimental methods for rapid screening of formulations
Experimental methods complement modelling approaches for chemical product formulation by providing data for model validation and parameter estimation, and insights into complex phenomena that can reveal incorrect assumptions or missing mechanisms in a model.
Several novel rheological methods are emerging in academic labs and on the market
A long-standing bottleneck in predicting the performance of a formulated product is that the analytical instruments available in the R&D phase may not correctly reproduce the realistic flow conditions during processing (Figure 3). Processing flows can exhibit large fluid velocities in small gaps and can be highly unsteady due to turbulence or during start-up. State-of-the-art characterizations such as spectroscopy, optical and electron microscopy, provide essential data on the composition, structure, and morphology of materials. However, this information is insufficient for predicting formulation behavior under flow, due to the dynamic changes in microstructure caused by flow and deformation.
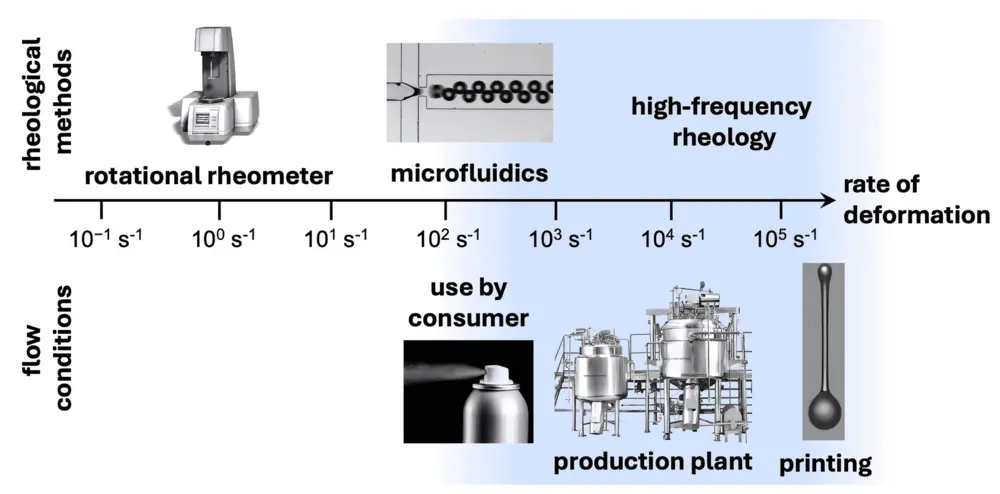
Image of droplet in ink-jet printing process reproduced from Phys. Rev. Appl. 1, 014004 (2014)
Rheological measurements, including viscosity, shear modulus, and yield stress, offer insights into the flow behavior of formulations and experimental data for validating CFD models and optimizing processing conditions. Experimental measurements of rheological properties in process-relevant conditions are however difficult. The available experimental methods, for instance rotational rheometers and microfluidics, cannot access the typical flow conditions of a processing flow, while the microstructure and properties of a formulated product are highly dependent on the flow conditions. Several novel rheological methods are emerging in academic labs and on the market, to extend the range of flow conditions beyond what is possible with conventional rheometers, based for instance on pressure-driven capillary flows, piezoelectric actuators, and ultrasound-mediated deformations.
Another direction for development of experimental methods is synergistic with the emergence of data-driven approaches, which require multi-dimensional datasets from a large number of samples and ideally also in different flow conditions. To enable this development, high-throughput experimental methods are desirable. Traditional rheometers can now be equipped with robotic handling of samples for high-throughput experimentations, and there is ample room for disruptive innovations in experimental methods that can provide massively parallel screening of samples.
Advancing models and experimental methods for virtual screening
In conclusion, multi-scale, multi-fidelity modelling approaches supported by novel experimental methods for rapid screening in process-relevant conditions will accelerate chemical product formulation and re-formulation, and the successful commercialization of new chemical products. Empirical, mechanistic, and AI-driven modelling techniques offer complementary tools for optimizing formulations. Formulating products for circularity and recyclability also requires consideration of material properties and process compatibility.
More broadly, challenges in sustainable chemical product formulation also encompass balancing performance, cost, and environmental impact. Overcoming these challenges will require interdisciplinary collaboration, and close partnerships between industry and research organizations. Regulatory alignment and consumer demand for eco-friendly alternatives further shape the landscape, driving the chemical industry towards greener and more sustainable practices.
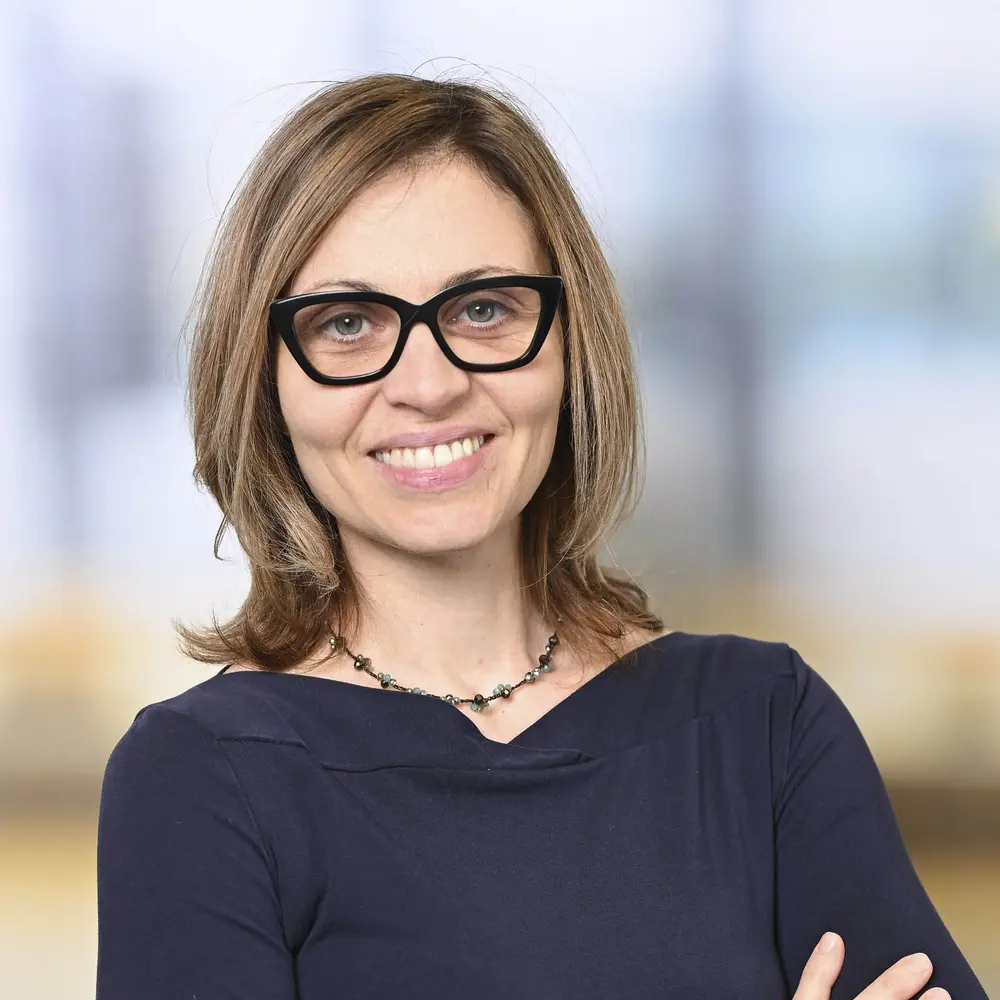
Valeria Garbin is Professor of Flow and Dynamics of Soft Matter in the Department of Chemical Engineering at Delft University of Technology (TU Delft). In her research program she integrates fluid dynamics with soft matter physics and colloid & interface science, to accurately understand the flow phenomena of complex, microstructured fluids and soft materials. This fundamental expertise is applied to the development of sustainable products and processes, as part of the transition to a circular economy.