We all need “Feedback”
The way to control processes is to close the loop
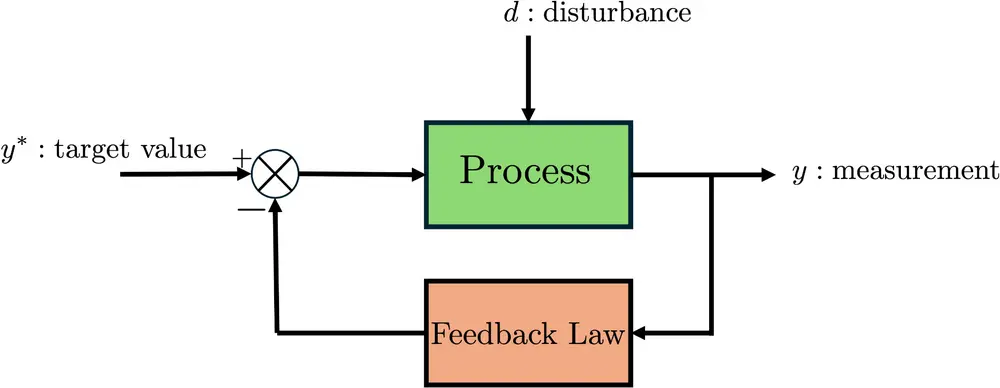
The world is changing rapidly, and these changes also affect chemical processing plants. Therefore, we approach the modeling and operation of these processes in an alternative way. This allows us to incorporate variables like energy availability, or optimize for minimum exhaust gasses in addition to economic value.
Uncertain World
We live in an uncertain world that is getting more uncertain every day. Markets get more dynamic and energy availability is less predictable. This growing unpredictability affects operations in various industries, including the process industry; uncertainty in the process industry appears in the form of variations in feedstock, production planning and energy sources, but also due to limited understanding of physical phenomena, for example, fouling.
Dealing with variations while enabling systems to operate in a desired manner has been the central theme of automatic control. Feedback (Figure 1), in particular, is the principal control mechanism that corrects for the error but also reduces the effect of uncertainty on the system behavior.
"We believe that chemical processes must be agile to dynamic condition"
Automatic control, which dates back to the 1800s, shares common features with Process Systems Engineering. Both approaches use systems thinking, and in the case of automatic control, this takes the form of dynamic concepts such as interconnections, process networks, feedback, and delay [1].
At the Smart Process Operations and Control Lab (SPROC) of the Control Systems Group at the Electrical Engineering Department of TU/e, we combine our knowledge of chemical and electrical engineering, system theory and control, and applied mathematics to address the operational challenges of the process industry (Figure 2).
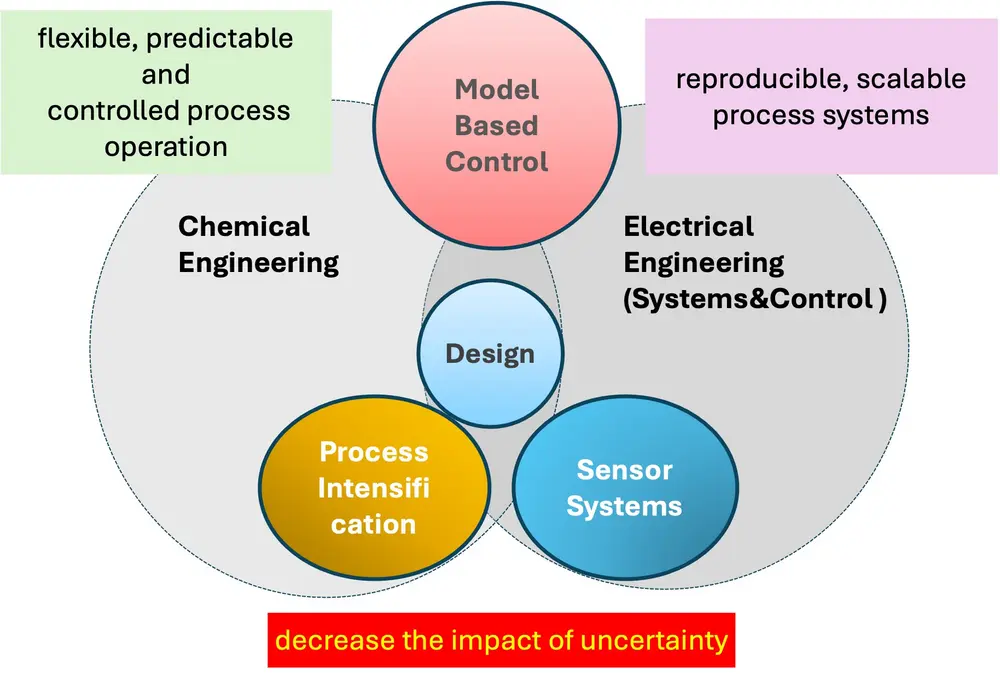
The SPROC way of working
Traditionally, chemical processes are designed and operated around economically efficient steady-state conditions. Nowadays, there is a drive to achieve sustainability goals by circularity and electrification using renewables.
The impact of this drive on the operations is that there is no longer a single optimal operating condition. Instead, we are left with a choice of optimal trajectories. Therefore, we believe that chemical processes must be agile to dynamic conditions, such as varying production goals in relation to the availability of energy or due to interactions with other industrial sectors.
At SPROC, we focus on understanding dynamic process behavior and developing control technology to enhance the flexibility of process systems, all to drive the chemical processes to sustainable outcomes.
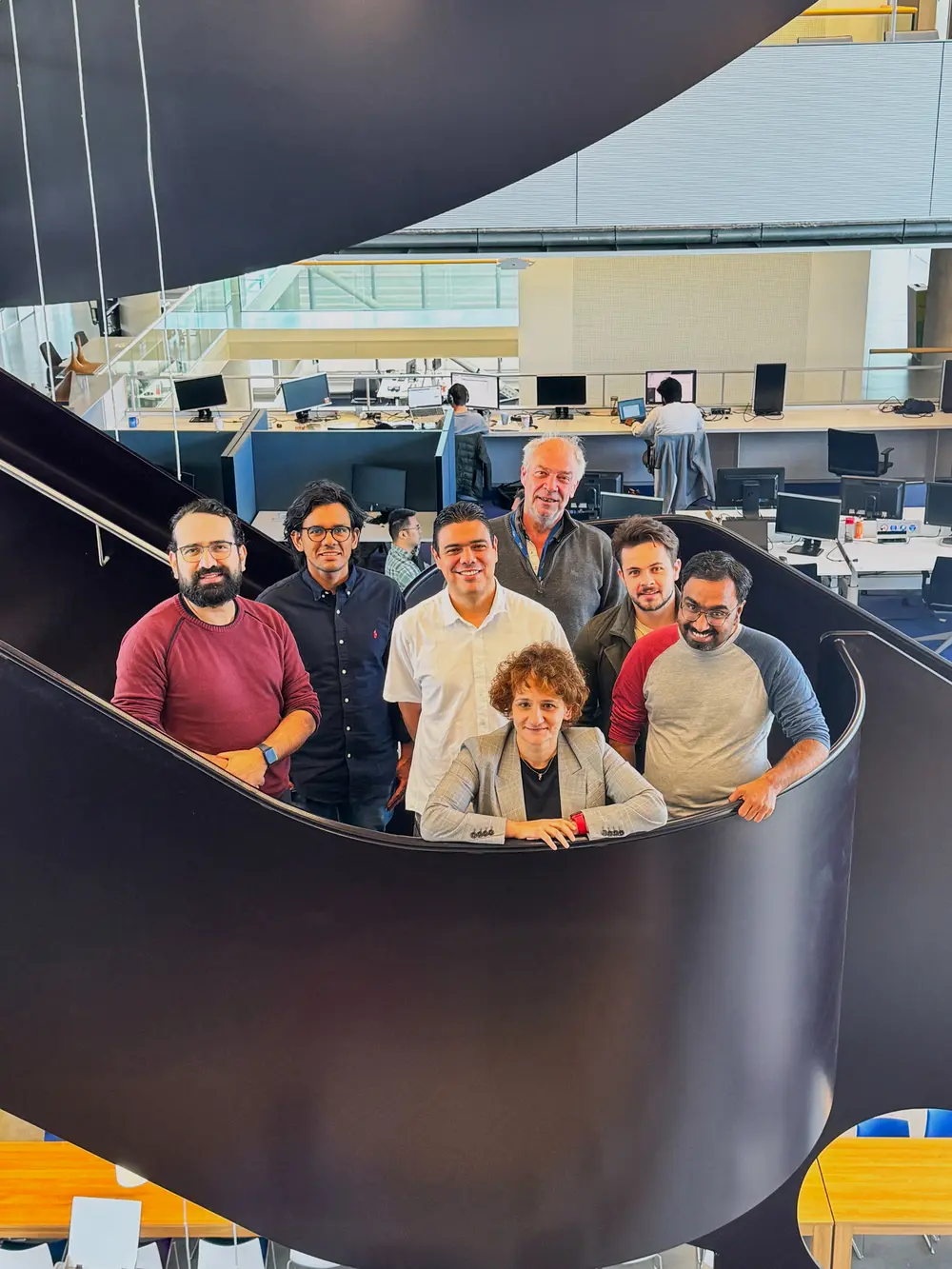
Understanding dynamic process behavior
The purpose of process dynamics is to study how time and external factors affect the process. It analyzes the response time it takes for the process to external excitation and the type of this response, whether it is with some delay or it reaches steady state conditions.
It also enables us to analyze the degrees of freedom and select them in a manner that maximizes their potential impact on the process behavior.
"We study how to develop process dynamic models suitable for real-time control algorithms"
This way of working is more than analyzing a set of differential equations but requires us to think carefully about the underlying physics and operational restrictions. Chemical processes have inertia and, therefore, cannot just change the quality and quantity of their products instantaneously. Using control technology and feedback mechanisms, we can steer chemical processes to the desired dynamic behavior while reducing the impact of uncertainty, which is what we want. However, we are still dependent on our sensors. But we have ways of dealing with that challenge.
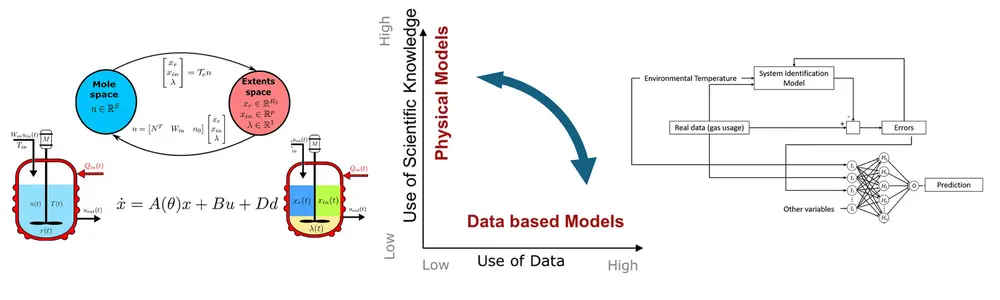
Modeling for Control
Mathematical models are extensively used in engineering disciplines for different purposes. In PSE, models are used for flowsheet optimization, process optimization, life cycle assessment, and many other applications.
Mathematical modeling is also the tool our team uses to describe process dynamics. In automatic control, linear and data-driven models have been successfully utilized to develop control algorithms. However, these models have limited validity, are local to a specific operating point, and definitely do not own the predictive capability required for flexible process operation.
Detailed models based on physical laws can be used, but they are still expensive to develop, maintain and computationally demanding for online applications like control. At SPROC, we study how to develop process dynamic models suitable for real-time control algorithms.
Model reduction, shortcut methods, and hybrid modeling (combining physical models with data-driven models like machine learning, Figure 3) are a few examples of approaches we study and investigate their suitability for control [5].
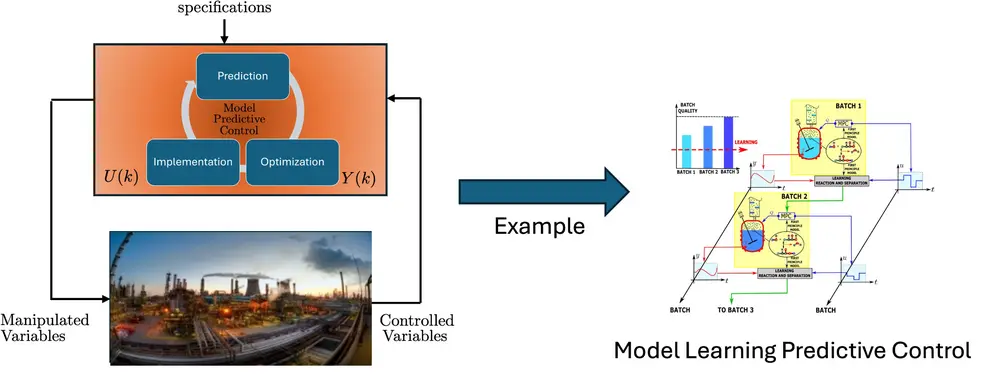
Controlling Dynamic Behavior
Understanding dynamics through models guides us, when we are developing model-based control technology. Model predictive control (Figure 4), for example, with linear models, is an accepted and proven technology for the process industry. The philosophy of this control strategy keeps process systems close to their constraints while still optimizing for maximum economic value.
However, the commissioning of such a controller requires a certain skill set. And the lifetime performance of such technology is limited due to a lack of autonomous maintenance [2].
Instead at SPROC, we work on bringing this control technology to a high level of autonomy. Moreover, we need additional technical developments such as i) computationally tractable formulations to deal with ever-growing uncertainty in process systems and with nonlinear models ii) formulations that can interact with the other layers of the control hierarchy like planning and scheduling [4, 3]
The latter is becoming very relevant since the shift to green electricity will make the supply of electricity more dynamic. That means the chemical industry will need to adapt and change their processes to operate more dynamically. Most importantly, process technology should lead to fully predictable production processes and volumes and be transparent to process engineers and operators .
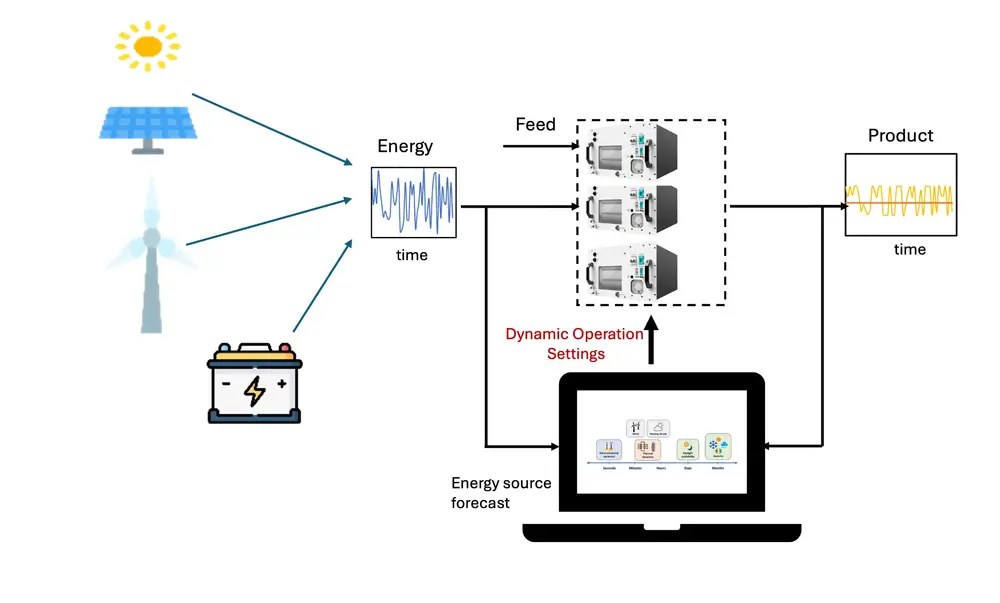
(Re)designing processes and process control
How we design chemical processes has consequences for the process dynamics and control strategies. The selection of actuators and sensors, combined with the sizing of unit operations and underlying physics, decides the process's dynamic behavior. Traditionally, a sequential approach is implemented.
First, the process design is executed, followed by the control design. Such a strategy results in suboptimal process dynamic behavior and, in some cases, the inability to steer the process to a desired operating condition.
Several times, it has been demonstrated that control structure selection at the process design stage improves the closed-loop performance. Still, it is a very complex problem to solve.
This problem is getting even more complex now since electrification initiatives require agile processes that can operate dynamically and control technology that can enable this (Figure 5).
That’s why feedback saves the process
With all the new initiatives and drivers, we are, in a way, applying feedback to correct the shortcomings and errors of the past. This is essential, but what is more essential is to anticipate and take feedforward action for a sustainable future.
References:
[1] P.P, Khargonekar, T. Samad, The United Nations Sustainable Development Gioals: An IFAC Agenda, IFAC PapersOnLine 58, 153-158, 2024
[2] L. Ozkan, et.al. Advanced autonomous model-based operation of industrial process systems (Autoprofit): Technological Developments and Future perspectives, Annual Reviews in Control, 42, p. 126-142, 2016
[3] M. B. Saltik et.al., Moment Based Model Predictive Control for Linear Systems: Additive perturbations case, Int. J. Robust and Nonlinear Control, 32, 15, 0. 8252-8279
[4] R. Dirza, et.al., Integration of max-plus lienar scheduling and control, computer Aided Chemcial Engineering, 46, 1279-1284, 2019
[5] Akan.T et. al. Model Discrepancy Learning for HEX Networks, IFAC PapersOnline, 58, 14, 403-406, 2024